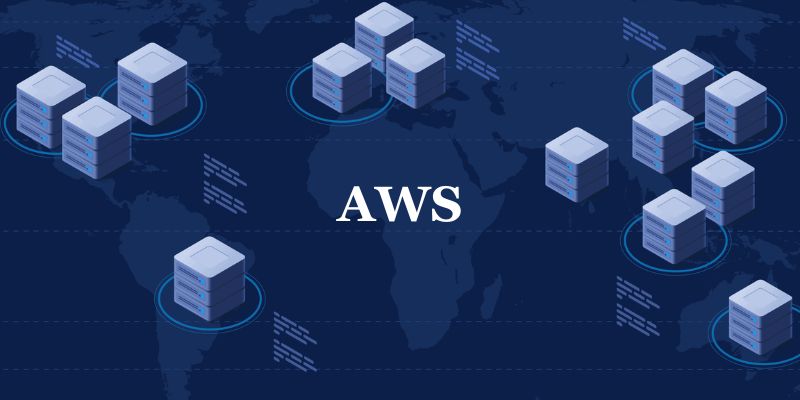
In today’s data-driven world, machine learning (ML) has become a crucial tool for businesses seeking to gain insights, automate processes, and drive innovation. However, implementing ML models can be complex and resource-intensive. This is where Amazon Web Services (AWS) SageMaker comes into play. SageMaker is a fully managed service that simplifies the process of building, training, and deploying ML models at scale, making it an ideal choice for organizations looking to leverage the power of ML effectively. Institutions offering AWS Training in Chennai recognize the growing demand for professionals skilled in AWS services like SageMaker. Let’s explore how organizations can harness the power of machine learning with AWS SageMaker.
Power of Machine Learning with AWS
Introduction to AWS SageMaker
AWS SageMaker is a comprehensive platform that provides developers and data scientists with the tools they need to build, train, and deploy ML models quickly and efficiently. It offers a wide range of features, including built-in algorithms, automated model tuning, and seamless integration with other AWS services.
Data Preparation and Exploration
Before building an ML model, it’s essential to prepare and explore the data thoroughly. SageMaker provides tools for data preprocessing, visualization, and analysis, allowing users to gain insights into their datasets and identify patterns that can inform model development.
Model Building and Training
With SageMaker, building and training ML models is streamlined and accessible to users of all skill levels. The platform offers a variety of built-in algorithms and frameworks, as well as support for custom code, enabling developers to choose the approach that best suits their needs. Institutions offering an AWS Course at FITA Academy understand the importance of SageMaker in providing hands-on experience with building and training ML models on the AWS platform. Additionally, SageMaker’s automatic model tuning feature helps optimize model performance by fine-tuning hyperparameters.
Deployment and Scaling
Once a model is trained, SageMaker makes it easy to deploy it into production environments with just a few clicks. The platform supports various deployment options, including real-time inference endpoints and batch transformations, ensuring that models can meet the demands of any application. Furthermore, SageMaker’s auto-scaling capabilities allow organizations to handle fluctuating workloads efficiently.
Monitoring and Management
Monitoring the performance of deployed ML models is critical for ensuring reliability and accuracy. SageMaker provides built-in monitoring capabilities that enable users to track key metrics, detect anomalies, and set up alerts for potential issues. Additionally, the platform offers comprehensive management tools for versioning models, managing resources, and controlling access.
Cost Optimization
Cost management is a key consideration for any ML project. SageMaker helps organizations optimize their ML workflows by offering pay-as-you-go pricing, which means users only pay for the resources they consume. Additionally, the platform provides tools for resource utilization analysis and cost estimation, allowing organizations to make informed decisions about resource allocation.
AWS SageMaker empowers organizations to unlock the full potential of machine learning by providing a comprehensive and scalable platform for model development, training, deployment, and management. Institutions offering AWS Training in Bangalore recognize the significance of SageMaker in preparing professionals to leverage AWS services effectively. With its robust features and seamless integration with other AWS services, SageMaker simplifies the ML lifecycle and enables organizations to innovate faster and more effectively. By harnessing the power of machine learning with AWS SageMaker, businesses can drive greater efficiency, improve decision-making, and unlock new opportunities for growth and innovation.
Also Check: Enhancing Cloud Networking with AWS Transit Gateway